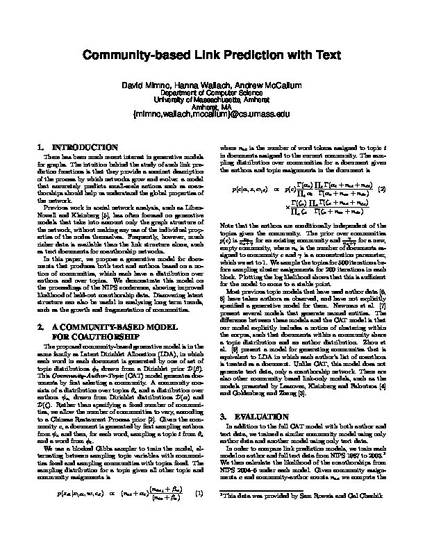
Unpublished Paper
Community-based Link Prediction with Text
(2007)
Abstract
There has been much recent interest in generative models for graphs. The intuition behind the study of such link prediction functions is that they provide a succinct description of the process by which networks grow and evolve: a model that accurately predicts small-scale actions such as coauthorships should help us understand the global properties of the network.
Previous work in social network analysis, such as Liben-Nowell and Kleinberg [5], has often focused on generative models that take into account only the graph structure of the network, without making any use of the individual properties of the nodes themselves. Frequently, however, much richer data is available than the link structure alone, such as text documents for coauthorship networks.
In this paper, we propose a generative model for documents that produces both text and authors based on a notion of communities, which each have a distribution over authors and over topics. We demonstrate this model on the proceedings of the NIPS conference, showing improved likelihood of held-out coauthorship data. Discovering latent structure can also be useful in analyzing long term trends, such as the growth and fragmentation of communities.
Disciplines
Publication Date
2007
Comments
This is the pre-published version harvested from CIIR.
Citation Information
David Mimno, Hanna M. Wallach and Andrew McCallum. "Community-based Link Prediction with Text" (2007) Available at: http://works.bepress.com/hanna_wallach/2/