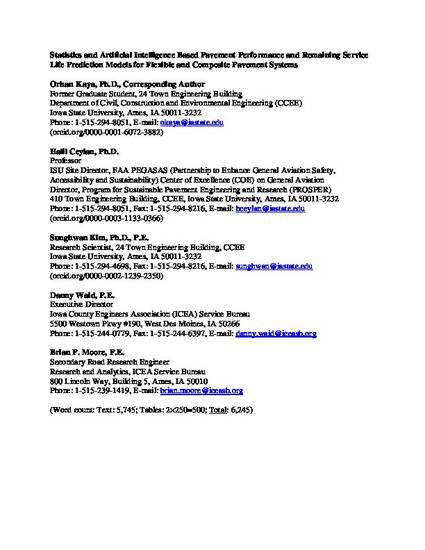
In their pavement management decision-making processes, U.S. state highway agencies are required to develop performance-based approaches by the Moving Ahead for Progress in the 21st Century (MAP-21) federal transportation legislation. One of the performance-based approaches to facilitate pavement management decision-making processes is the use of remaining service life (RSL) models. In this study, a detailed step-by-step methodology for the development of pavement performance and RSL prediction models for flexible and composite (asphalt concrete [AC] over jointed plain concrete pavement [JPCP]) pavement systems in Iowa is described. To develop such RSL models, pavement performance models based on statistics and artificial intelligence (AI) techniques were initially developed. While statistically defined pavement performance models were found to be accurate in predicting pavement performance at project level, AI-based pavement performance models were found to be successful in predicting pavement performance in network level analysis. Network level pavement performance models using both statistics and AI-based approaches were also developed to evaluate the relative success of these two models for network level pavement performance modeling. As part of this study, in the development of pavement RSL prediction models, automation tools for future pavement performance predictions were developed and used along with the threshold limits for various pavement performance indicators specified by the Federal Highway Administration. These RSL models will help engineers in decision-making processes at both network and project levels and for different types of pavement management business decisions.
Available at: http://works.bepress.com/halil_ceylan/339/
This is a manuscript of an article published as Kaya, Orhan, Halil Ceylan, Sunghwan Kim, Danny Waid, and Brian P. Moore. "Statistics and Artificial Intelligence-Based Pavement Performance and Remaining Service Life Prediction Models for Flexible and Composite Pavement Systems." Transportation Research Record (2020). DOI: 10.1177%2F0361198120915889. Reprinted by permission of SAGE Publications.