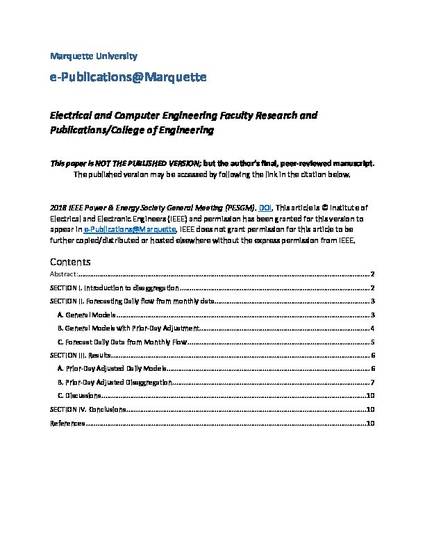
Many needs exist in the energy industry where measurement is monthly yet daily values are required. The process of disaggregation of low frequency measurement to higher frequency values has been presented in this literature. Also, a novel method that accounts for prior-day weather impacts in the disaggregation process is presented, even though prior-day impacts are not directly recoverable from monthly data. Having initial daily weather and gas flow data, the weather and flow data are aggregated to generate simulated monthly weather and consumption data. Linear regression models can be powerful tools for parametrization of monthly/daily consumption models and will enable accurate disaggregation. Two-, three-, four-, and six-parameter linear regression models are built. RMSE and MAPE are used as means for assessing the performance of the proposed approach. Extensive comparisons between the monthly/daily gas consumption forecasts show higher accuracy of the results when the effect of prior-day weather inputs are considered.
Available at: http://works.bepress.com/george_corliss/22/
Accepted version. 2018 IEEE Power & Energy Society General Meeting (PESGM), (August 5-10, 2018). DOI. © Institute of Electrical and Electronic Engineers (IEEE). Used with permission.