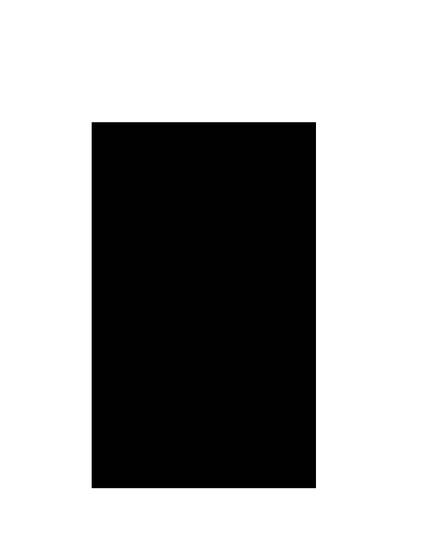
We model sequential, possibly multiunit, sealed bid auctions as a sequential game with imperfect and incomplete information. We develop an agent that constructs a bidding policy by sampling the valuation space of its opponents, solving the resulting complete information game, and aggregating the samples into a policy. The constructed policy takes advantage of information learned in the early stages of the game and is flexible with respect to assumptions about the other bidders' valuations. Because the straightforward expansion of the complete information game is intractable, we develop a more concise representation that takes advantage of the sequential auctions' natural structure. We examine the performance of our agent versus agents that play perfectly, agents that also create policies using Monte Carlo, and other benchmarks. The technique performs quite well in these empirical studies, although the tractability of the problem is bounded by the ability to solve component games.
© 2005. This manuscript version is made available under the CC-BY-NC-ND 4.0 license https://creativecommons.org/licenses/by-nc-nd/4.0/