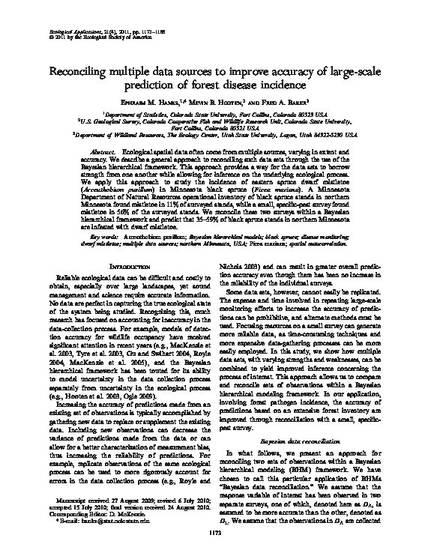
Article
Reconciling multiple data sources to improve accuracy of large-scale prediction of forest disease incidence
Ecological Applications
(2011)
Abstract
Ecological spatial data often come from multiple sources, varying in extent and accuracy. We describe a general approach to reconciling such data sets through the use of the Bayesian hierarchical framework. This approach provides a way for the data sets to borrow strength from one another while allowing for inference on the underlying ecological process. We apply this approach to study the incidence of eastern spruce dwarf mistletoe (Arceuthobium pusillum) in Minnesota black spruce (Picea mariana). A Minnesota Department of Natural Resources operational inventory of black spruce stands in northern Minnesota found mistletoe in 11% of surveyed stands, while a small, specific-pest survey found mistletoe in 56% of the surveyed stands. We reconcile these two surveys within a Bayesian hierarchical framework and predict that 35-59% of black spruce stands in northern Minnesota are infested with dwarf mistletoe.
Keywords
- Arceuthobium pusillum; Bayesian hierarchical models; black spruce; disease monitoring; dwarf mistletoe; multiple data sources; northern Minnesota,
- USA; Picea mariana; spatial autocorrelation
Disciplines
Publication Date
June, 2011
DOI
https://doi.org/10.1890/09-1549.1
Publisher Statement
http://dx.doi.org/10.1890/09-1549.1
Citation Information
Fred A. Baker. "Reconciling multiple data sources to improve accuracy of large-scale prediction of forest disease incidence" Ecological Applications Vol. 21 Iss. 4 (2011) p. 1173 - 1188 Available at: http://works.bepress.com/fred_baker/10/