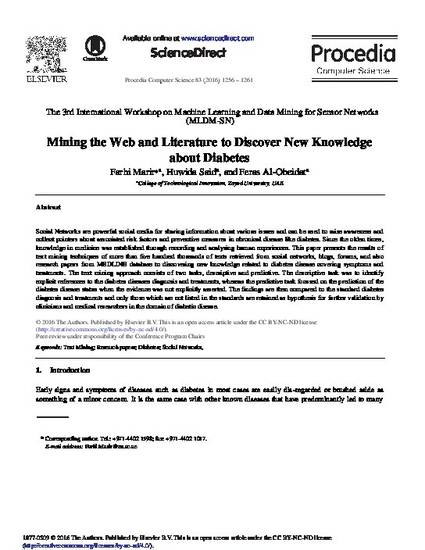
© 2016 The Authors. Social Networks are powerful social media for sharing information about various issues and can be used to raise awareness and collect pointers about associated risk factors and preventive measures in chronical disease like diabetes. Since the olden times, knowledge in medicine was established through recording and analysing human experiences. This paper presents the results of text mining techniques of more than five hundred thousands of texts retrieved from social networks, blogs, forums, and also research papers from MEDLINE database to discovering new knowledge related to diabetes disease covering symptoms and treatments. The text mining approach consists of two tasks, descriptive and predictive. The descriptive task was to identify explicit references to the diabetes diseases diagnosis and treatments, whereas the predictive task focused on the prediction of the diabetes disease status when the evidence was not explicitly asserted. The findings are then compared to the standard diabetes diagnosis and treatments and only those which are not listed in the standards are retained as hypothesis for further validation by clinicians and medical researchers in the domain of diabetic disease.
- Diabetes,
- Research papers,
- Social Networks,
- Text Mining
Available at: http://works.bepress.com/feras-al-obeidat/37/