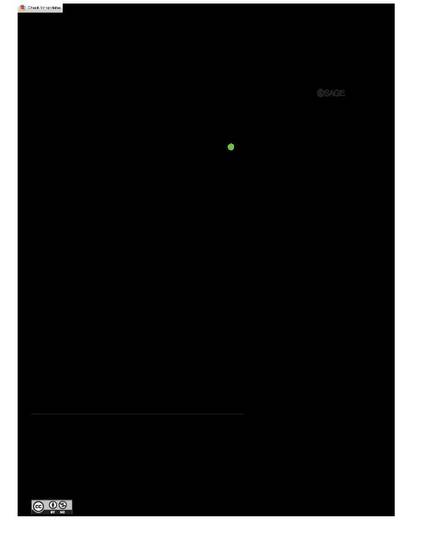
© The Author(s) 2018. A novel framework for the classification of lung nodules using computed tomography scans is proposed in this article. To get an accurate diagnosis of the detected lung nodules, the proposed framework integrates the following 2 groups of features: (1) appearance features modeled using the higher order Markov Gibbs random field model that has the ability to describe the spatial inhomogeneities inside the lung nodule and (2) geometric features that describe the shape geometry of the lung nodules. The novelty of this article is to accurately model the appearance of the detected lung nodules using a new developed seventh-order Markov Gibbs random field model that has the ability to model the existing spatial inhomogeneities for both small and large detected lung nodules, in addition to the integration with the extracted geometric features. Finally, a deep autoencoder classifier is fed by the above 2 feature groups to distinguish between the malignant and benign nodules. To evaluate the proposed framework, we used the publicly available data from the Lung Image Database Consortium. We used a total of 727 nodules that were collected from 467 patients. The proposed system demonstrates the promise to be a valuable tool for the detection of lung cancer evidenced by achieving a nodule classification accuracy of 91.20%.
- Autoencoder,
- Computed tomography,
- Computer-aided diagnosis,
- Higher order MGRF,
- Lung cancer,
- Pulmonary nodule
Available at: http://works.bepress.com/fatma-taher/30/