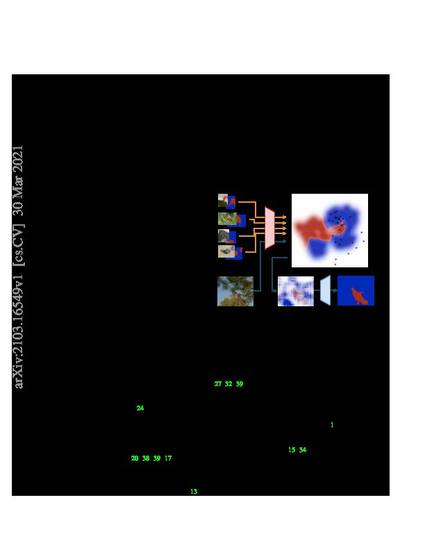
Few-shot segmentation is a challenging task, requiring the extraction of a generalizable representation from only a few annotated samples, in order to segment novel query images. A common approach is to model each class with a single prototype. While conceptually simple, these methods suffer when the target appearance distribution is multi-modal or not linearly separable in feature space. To tackle this issue, we propose a few-shot learner formulation based on Gaussian process (GP) regression. Through the expressivity of the GP, our approach is capable of modeling complex appearance distributions in the deep feature space. The GP provides a principled way of capturing uncertainty, which serves as another powerful cue for the final segmentation, obtained by a CNN decoder. We further exploit the end-to-end learning capabilities of our approach to learn the output space of the GP learner, ensuring a richer encoding of the segmentation mask. We perform comprehensive experimental analysis of our few-shot learner formulation. Our approach sets a new state-of-the-art for 5-shot segmentation, with mIoU scores of 68.1 and 49.8 on PASCAL-5i and COCO-20i, respectively. © 2021, CC BY-NC-SA.
- Computer Vision and Pattern Recognition (cs.CV)
"Preprint: arXiv
Archived with thanks to arXiv
Preprint License: CC BY-NC-SA 4.0
Uploaded 24 March 2022"