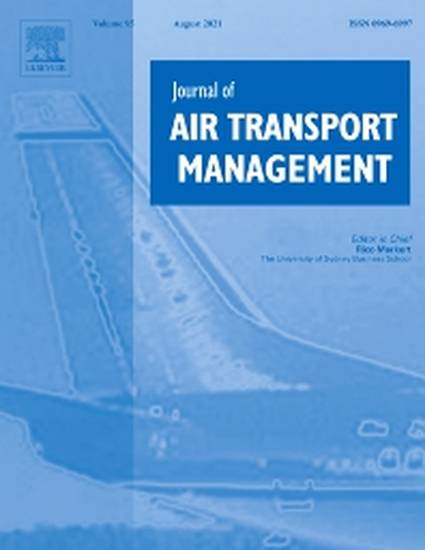
Article
Using Causal Machine Learning for Predicting the Risk of Flight Delays in Air Transportation
Journal of Air Transport Management
(2021)
Abstract
Delays in air transportation are a major concern that has negative impacts on the airline industry and the economy. Given the complexity of the National Air Space system, predicting the risk of flight delays and identifying significant predictors is vital to risk mitigation. The purpose of this paper is to perform data mining using causal machine learning algorithms in the USELEI process (Understanding, Sampling, Exploring, Learning, Evaluating, and Inferring) to predict the probability of flight delays in air transportation using data collected from different sources. The findings indicated significant effects of predictors, including reported arrivals and departures, arrival and departure demands, capacity, efficiency, and traffic volume at the origin and destination airports on the risk of flight delays. More importantly, causal interrelationships among variables in a fully structural network are presented to how these predictors interact with one another and how these interactions lead to delay incidents. Finally, sensitivity analysis and causal inference can be performed to evaluate various what-if scenarios and form effective strategies to mitigate the risk of delays.
Disciplines
Publication Date
March, 2021
DOI
https://doi.org/10.1016/j.jairtraman.2020.101993
Citation Information
Dothang Truong. "Using Causal Machine Learning for Predicting the Risk of Flight Delays in Air Transportation" Journal of Air Transport Management Vol. 91 (2021) Available at: http://works.bepress.com/dtruong/65/