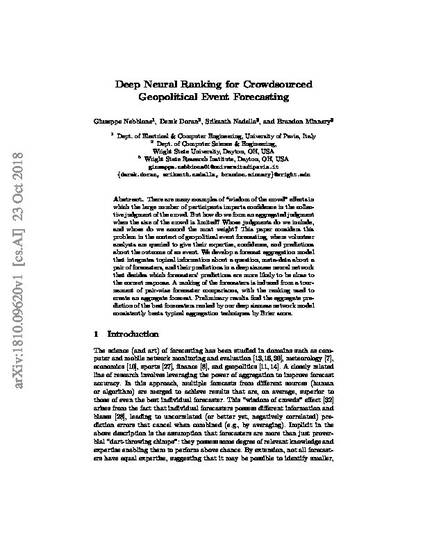
There are many examples of “wisdom of the crowd” effects in which the large number of participants imparts confidence in the collective judgment of the crowd. But how do we form an aggregated judgment when the size of the crowd is limited? Whose judgments do we include, and whose do we accord the most weight? This paper considers this problem in the context of geopolitical event forecasting, where volunteer analysts are queried to give their expertise, confidence, and predictions about the outcome of an event. We develop a forecast aggregation model that integrates topical information about a question, meta-data about a pair of forecasters, and their predictions in a deep siamese neural network that decides which forecasters’ predictions are more likely to be close to the correct response. A ranking of the forecasters is induced from a tournament of pair-wise forecaster comparisons, with the ranking used to create an aggregate forecast. Preliminary results find the aggregate prediction of the best forecasters ranked by our deep siamese network model consistently beats typical aggregation techniques by Brier score.
Available at: http://works.bepress.com/derek_doran/58/