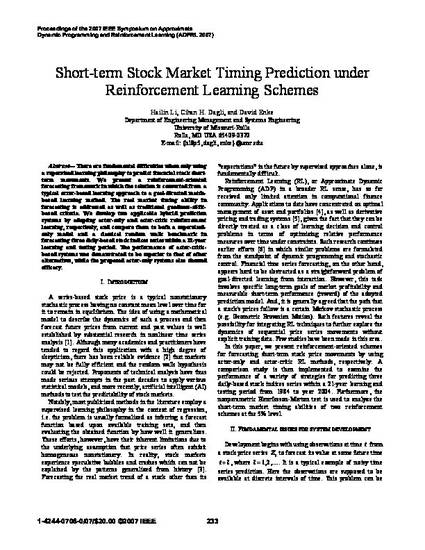
There are fundamental difficulties when only using a supervised learning philosophy to predict financial stock short-term movements. We present a reinforcement-oriented forecasting framework in which the solution is converted from a typical error-based learning approach to a goal-directed match-based learning method. The real market timing ability in forecasting is addressed as well as traditional goodness-of-fit-based criteria. We develop two applicable hybrid prediction systems by adopting actor-only and actor-critic reinforcement learning, respectively, and compare them to both a supervised-only model and a classical random walk benchmark in forecasting three daily-based stock indices series within a 21-year learning and testing period. The performance of actor-critic-based systems was demonstrated to be superior to that of other alternatives, while the proposed actor-only systems also showed efficacy
- Stock Market,
- Forecasting Framework,
- Random Walk Benchmark,
- Timing Prediction
Available at: http://works.bepress.com/david-enke/23/