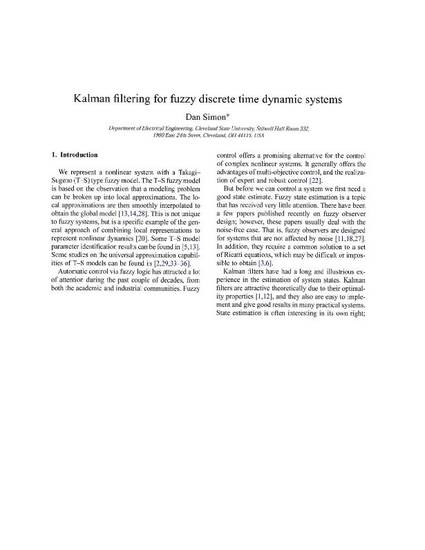
This paper uses Kalman filter theory to design a state estimator for noisy discrete time Takagi–Sugeno (T–S) fuzzy models. One local filter is designed for each local linear model using standard Kalman filter theory. Steady state solutions can be found for each of the local filters. Then a linear combination of the local filters is used to derive a global filter. The local filters are time-invariant, which greatly reduces the computational complexity of the global filter. The global filter is shown to be unbiased and (under certain conditions) stable. In addition, under the approximation of uncorrelatedness among the local models, the global filter is shown to be minimum variance. The proposed state estimator is demonstrated on a vehicle tracking problem and a backing up truck–trailer example.