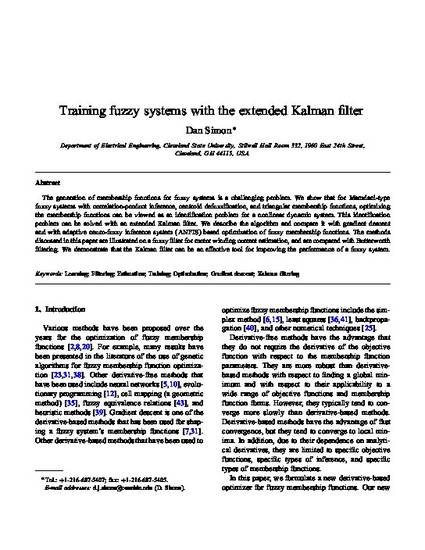
The generation of membership functions for fuzzy systems is a challenging problem. We show that for Mamdani-type fuzzy systems with correlation-product inference, centroid defuzzification, and triangular membership functions, optimizing the membership functions can be viewed as an identification problem for a nonlinear dynamic system. This identification problem can be solved with an extended Kalman filter. We describe the algorithm and compare it with gradient descent and with adaptive neuro-fuzzy inference system (ANFIS) based optimization of fuzzy membership functions. The methods discussed in this paper are illustrated on a fuzzy filter for motor winding current estimation, and are compared with Butterworth filtering. We demonstrate that the Kalman filter can be an effective tool for improving the performance of a fuzzy system.