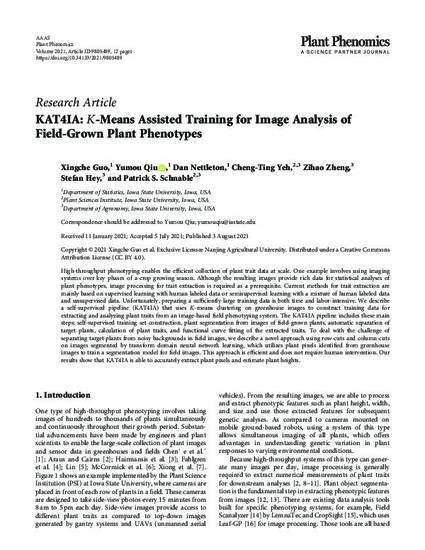
High-throughput phenotyping enables the efficient collection of plant trait data at scale. One example involves using imaging systems over key phases of a crop growing season. Although the resulting images provide rich data for statistical analyses of plant phenotypes, image processing for trait extraction is required as a prerequisite. Current methods for trait extraction are mainly based on supervised learning with human labeled data or semisupervised learning with a mixture of human labeled data and unsupervised data. Unfortunately, preparing a sufficiently large training data is both time and labor-intensive. We describe a self-supervised pipeline (KAT4IA) that uses -means clustering on greenhouse images to construct training data for extracting and analyzing plant traits from an image-based field phenotyping system. The KAT4IA pipeline includes these main steps: self-supervised training set construction, plant segmentation from images of field-grown plants, automatic separation of target plants, calculation of plant traits, and functional curve fitting of the extracted traits. To deal with the challenge of separating target plants from noisy backgrounds in field images, we describe a novel approach using row-cuts and column-cuts on images segmented by transform domain neural network learning, which utilizes plant pixels identified from greenhouse images to train a segmentation model for field images. This approach is efficient and does not require human intervention. Our results show that KAT4IA is able to accurately extract plant pixels and estimate plant heights.
Available at: http://works.bepress.com/dan-nettleton/136/
This article is published as Xingche Guo, Yumou Qiu, Dan Nettleton, Cheng-Ting Yeh, Zihao Zheng, Stefan Hey, Patrick S. Schnable, "KAT4IA: K-Means Assisted Training for Image Analysis of Field-Grown Plant Phenotypes", Plant Phenomics, vol. 2021, Article ID 9805489, 12 pages, 2021. doi:10.34133/2021/9805489.