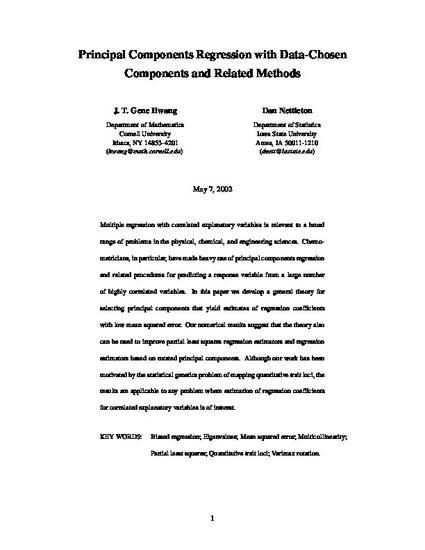
Multiple regression with correlated explanatory variables is relevant to a broad range of problems in the physical, chemical, and engineering sciences. Chemometricians in particular have made heavy use of principal components regression and related procedures for predicting a response variable from a large number of highly correlated variables. In this article we develop a general theory for selecting principal components that yield estimates of regression coefficients with low mean squared error. Our numerical results suggest that the theory also can be used to improve partial least squares regression estimators and regression estimators based on rotated principal components. Although our work has been motivated by the statistical genetics problem of mapping quantitative trait loci, the results are applicable to any problem in which estimation of regression coefficients for correlated explanatory variables is of interest.
Available at: http://works.bepress.com/dan-nettleton/126/
This is a manuscript of an article published as Hwang, JT Gene, and Dan Nettleton. "Principal components regression with data chosen components and related methods." Technometrics 45, no. 1 (2003): 70-79. doi: 10.1198/004017002188618716. Posted with permission.