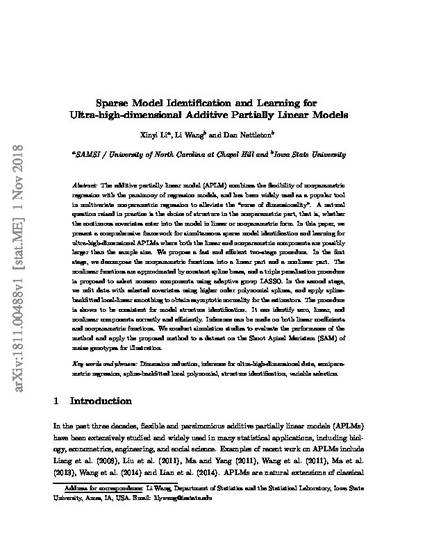
The additive partially linear model (APLM) combines the flexibility of nonparametric regression with the parsimony of regression models, and has been widely used as a popular tool in multivariate nonparametric regression to alleviate the “curse of dimensionality”. A natural question raised in practice is the choice of structure in the nonparametric part, i.e., whether the continuous covariates enter into the model in linear or nonparametric form. In this paper, we present a comprehensive framework for simultaneous sparse model identification and learning for ultra-high-dimensional APLMs where both the linear and nonparametric components are possibly larger than the sample size. We propose a fast and efficient two-stage procedure. In the first stage, we decompose the nonparametric functions into a linear part and a nonlinear part. The nonlinear functions are approximated by constant spline bases, and a triple penalization procedure is proposed to select nonzero components using adaptive group LASSO. In the second stage, we refit data with selected covariates using higher order polynomial splines, and apply spline-backfitted local-linear smoothing to obtain asymptotic normality for the estimators. The procedure is shown to be consistent for model structure identification. It can identify zero, linear, and nonlinear components correctly and efficiently. Inference can be made on both linear coefficients and nonparametric functions. We conduct simulation studies to evaluate the performance of the method and apply the proposed method to a dataset on the Shoot Apical Meristem (SAM) of maize genotypes for illustration.
Available at: http://works.bepress.com/dan-nettleton/122/
This is a manuscript of an article published as Li, Xinyi, Li Wang, and Dan Nettleton. "Sparse model identification and learning for ultra-high-dimensional additive partially linear models." Journal of Multivariate Analysis 173 (2019): 204-228. doi: 10.1016/j.jmva.2019.02.010. Posted with permission.