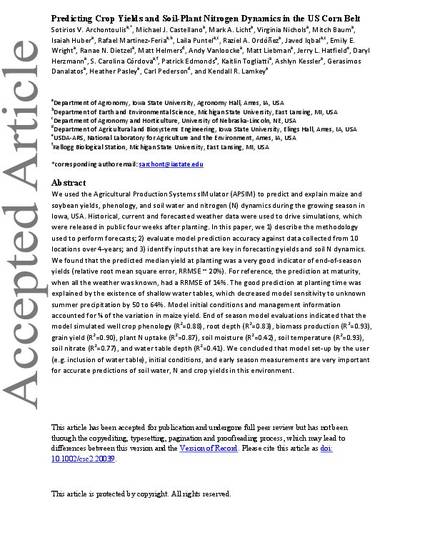
We used the Agricultural Production Systems sIMulator (APSIM) to predict and explain maize and soybean yields, phenology, and soil water and nitrogen (N) dynamics during the growing season in Iowa, USA. Historical, current and forecasted weather data were used to drive simulations, which were released in public four weeks after planting. In this paper, we (1) describe the methodology used to perform forecasts; (2) evaluate model prediction accuracy against data collected from 10 locations over four years; and (3) identify inputs that are key in forecasting yields and soil N dynamics. We found that the predicted median yield at planting was a very good indicator of end‐of‐season yields (relative root mean square error [RRMSE] of ∼20%). For reference, the prediction at maturity, when all the weather was known, had a RRMSE of 14%. The good prediction at planting time was explained by the existence of shallow water tables, which decreased model sensitivity to unknown summer precipitation by 50–64%. Model initial conditions and management information accounted for one‐fourth of the variation in maize yield. End of season model evaluations indicated that the model simulated well crop phenology (R2 = 0.88), root depth (R2 = 0.83), biomass production (R2 = 0.93), grain yield (R2 = 0.90), plant N uptake (R2 = 0.87), soil moisture (R2 = 0.42), soil temperature (R2 = 0.93), soil nitrate (R2 = 0.77), and water table depth (R2 = 0.41). We concluded that model set‐up by the user (e.g. inclusion of water table), initial conditions, and early season measurements are very important for accurate predictions of soil water, N and crop yields in this environment.
Available at: http://works.bepress.com/castellano-michael/56/
This article is published as Archontoulis, Sotirios V., Michael J. Castellano, Mark A. Licht, Virginia Nichols, Mitch Baum, Isaiah Huber, Rafael Martinez‐Feria et al. "Predicting crop yields and soil‐plant nitrogen dynamics in the US Corn Belt." Crop Science (2020). doi: 10.1002/csc2.20039.