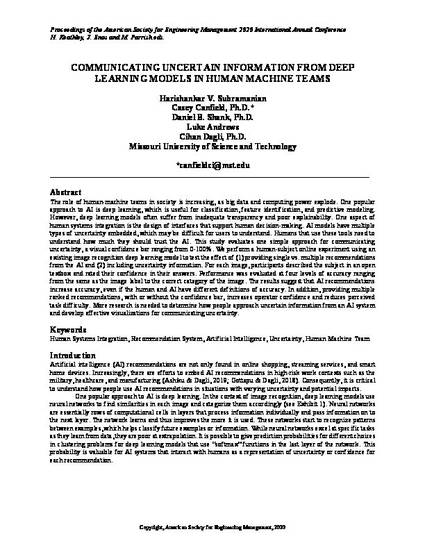
The role of human-machine teams in society is increasing, as big data and computing power explode. One popular approach to AI is deep learning, which is useful for classification, feature identification, and predictive modeling. However, deep learning models often suffer from inadequate transparency and poor explainability. One aspect of human systems integration is the design of interfaces that support human decision-making. AI models have multiple types of uncertainty embedded, which may be difficult for users to understand. Humans that use these tools need to understand how much they should trust the AI. This study evaluates one simple approach for communicating uncertainty, a visual confidence bar ranging from 0-100%. We perform a human-subject online experiment using an existing image recognition deep learning model to test the effect of (1) providing single vs. multiple recommendations from the AI and (2) including uncertainty information. For each image, participants described the subject in an open textbox and rated their confidence in their answers. Performance was evaluated at four levels of accuracy ranging from the same as the image label to the correct category of the image. The results suggest that AI recommendations increase accuracy, even if the human and AI have different definitions of accuracy. In addition, providing multiple ranked recommendations, with or without the confidence bar, increases operator confidence and reduces perceived task difficulty. More research is needed to determine how people approach uncertain information from an AI system and develop effective visualizations for communicating uncertainty.
- Human Systems Integration,
- Recommendation System,
- Artificial Intelligence,
- Uncertainty,
- Human Machine Team
Available at: http://works.bepress.com/casey-canfield/23/