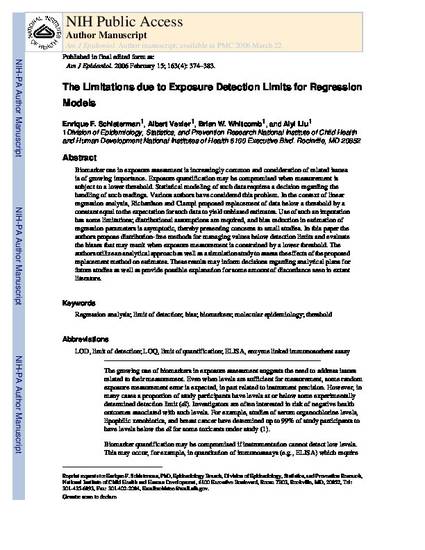
Article
The Limitations due to Exposure Detection Limits for Regression Models
American Journal of Epidemiology
(2006)
Abstract
Biomarker use in exposure assessment is increasingly common and consideration of related issues is of growing importance. Exposure quantification may be compromised when measurement is subject to a lower threshold. Statistical modeling of such data requires a decision regarding the handling of such readings. Various authors have considered this problem. In the context of linear regression analysis, Richardson and Ciampi proposed replacement of data below a threshold by a constant equal to the expectation for such data to yield unbiased estimates. Use of such an imputation has some limitations; distributional assumptions are required, and bias reduction in estimation of regression parameters is asymptotic, thereby presenting concerns to small studies. In this paper the authors propose distribution-free methods for managing values below detection limits and evaluate the biases that may result when exposure measurement is constrained by a lower threshold. The authors utilize an analytical approach as well as a simulation study to assess the effects of the proposed replacement method on estimates. These results may inform decisions regarding analytical plans for future studies as well as provide possible explanation for some amount of discordance seen in extant literature.
Disciplines
Publication Date
2006
Publisher Statement
The published version is located at http://www.ncbi.nlm.nih.gov/pmc/articles/PMC1408541/
Citation Information
Enrique F. Schisterman, Albert Vexler, Brian W Whitcomb and Aiyi Liu. "The Limitations due to Exposure Detection Limits for Regression Models" American Journal of Epidemiology Vol. 163 Iss. 4 (2006) Available at: http://works.bepress.com/brian_whitcomb/15/