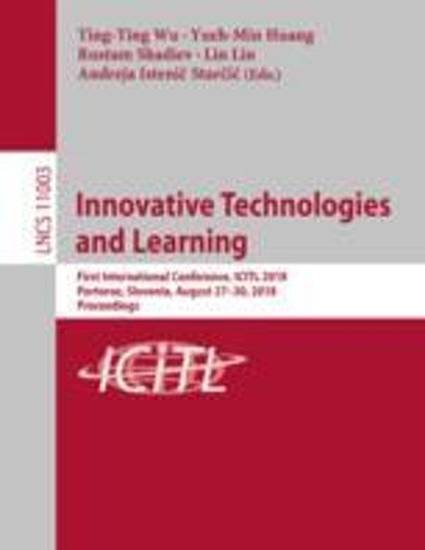
This study proposes an analytic approach which combines two predictive models (the predictive model of successful students and the predictive model of at-risk students) to enhance prediction performance for use under the constraints of limited data collection. A case study was conducted to examine the effects of the model combination approach. Eight variables were collected from a data warehouse and the Learning Management System. The best model was selected based on the lowest misclassification rate in the validation dataset. The confusion matrix compares the model’s performance with the following parameters: accuracy, misclassification, and sensitivity. The results show the new combination approach can capture more at-risk students than the singular predictive model, and is only suitable for the ensemble predictive algorithms.
Available at: http://works.bepress.com/brett_shelton/50/
Innovative Technologies and Learning: First International Conference, ICITL 2018, Portoroz, Slovenia, August 27–30, 2018, Proceedings is volume 11003 of the Lecture Notes in Computer Science book series