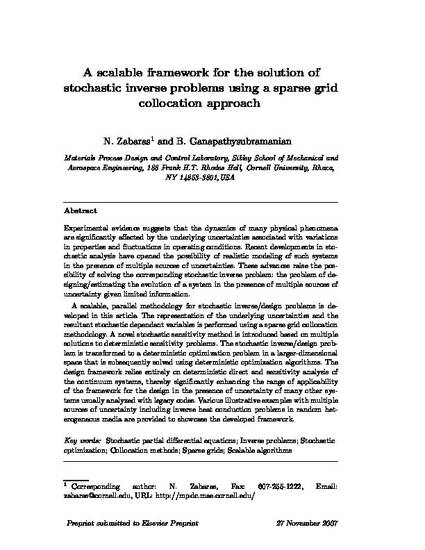
Article
A scalable framework for the solution of stochastic inverse problems using a sparse grid collocation approach
Journal of Computational Physics
(2008)
Abstract
Experimental evidence suggests that the dynamics of many physical phenomena are significantly affected by the underlying uncertainties associated with variations in properties and fluctuations in operating conditions. Recent developments in stochastic analysis have opened the possibility of realistic modeling of such systems in the presence of multiple sources of uncertainties. These advances raise the possibility of solving the corresponding stochastic inverse problem: the problem of designing/estimating the evolution of a system in the presence of multiple sources of uncertainty given limited information.
A scalable, parallel methodology for stochastic inverse/design problems is developed in this article. The representation of the underlying uncertainties and the resultant stochastic dependant variables is performed using a sparse grid collocation methodology. A novel stochastic sensitivity method is introduced based on multiple solutions to deterministic sensitivity problems. The stochastic inverse/design problem is transformed to a deterministic optimization problem in a larger-dimensional space that is subsequently solved using deterministic optimization algorithms. The design framework relies entirely on deterministic direct and sensitivity analysis of the continuum systems, thereby significantly enhancing the range of applicability of the framework for the design in the presence of uncertainty of many other systems usually analyzed with legacy codes. Various illustrative examples with multiple sources of uncertainty including inverse heat conduction problems in random heterogeneous media are provided to showcase the developed framework.
Keywords
- Stochastic partial differential equations,
- Sparse grids,
- Inverse problems,
- Stochastic optimization,
- Collocation methods,
- Scalable algorithms
Disciplines
Publication Date
April, 2008
DOI
10.1016/j.jcp.2008.01.019
Publisher Statement
This is a manuscript of an article from Journal of Computational Physics 227 (2008): 4697, doi:10.1016/j.jcp.2008.01.019. Posted with permission. Copyright 2008 Elsevier Inc.
Citation Information
Nicholas Zabaras and Baskar Ganapathysubramanian. "A scalable framework for the solution of stochastic inverse problems using a sparse grid collocation approach" Journal of Computational Physics Vol. 227 Iss. 9 (2008) p. 4697 - 4735 Available at: http://works.bepress.com/baskar-ganapathysubramanian/34/
Creative Commons license

This work is licensed under a Creative Commons CC_BY-NC-ND International License.