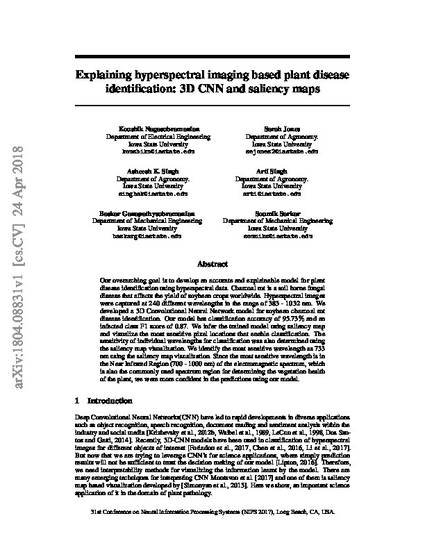
Our overarching goal is to develop an accurate and explainable model for plant disease identification using hyperspectral data. Charcoal rot is a soil borne fungal disease that affects the yield of soybean crops worldwide. Hyperspectral images were captured at 240 different wavelengths in the range of 383 - 1032 nm. We developed a 3D Convolutional Neural Network model for soybean charcoal rot disease identification. Our model has classification accuracy of 95.73\% and an infected class F1 score of 0.87. We infer the trained model using saliency map and visualize the most sensitive pixel locations that enable classification. The sensitivity of individual wavelengths for classification was also determined using the saliency map visualization. We identify the most sensitive wavelength as 733 nm using the saliency map visualization. Since the most sensitive wavelength is in the Near Infrared Region(700 - 1000 nm) of the electromagnetic spectrum, which is also the commonly used spectrum region for determining the vegetation health of the plant, we were more confident in the predictions using our model.
Available at: http://works.bepress.com/asheesh-singh/35/
This is a pre-print made available through arxiv: https://arxiv.org/abs/1804.08831.