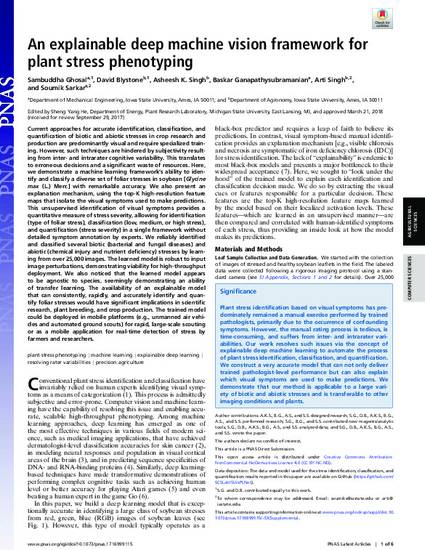
Current approaches for accurate identification, classification, and quantification of biotic and abiotic stresses in crop research and production are predominantly visual and require specialized training. However, such techniques are hindered by subjectivity resulting from inter- and intrarater cognitive variability. This translates to erroneous decisions and a significant waste of resources. Here, we demonstrate a machine learning framework’s ability to identify and classify a diverse set of foliar stresses in soybean [Glycine max (L.) Merr.] with remarkable accuracy. We also present an explanation mechanism, using the top-K high-resolution feature maps that isolate the visual symptoms used to make predictions. This unsupervised identification of visual symptoms provides a quantitative measure of stress severity, allowing for identification (type of foliar stress), classification (low, medium, or high stress), and quantification (stress severity) in a single framework without detailed symptom annotation by experts. We reliably identified and classified several biotic (bacterial and fungal diseases) and abiotic (chemical injury and nutrient deficiency) stresses by learning from over 25,000 images. The learned model is robust to input image perturbations, demonstrating viability for high-throughput deployment. We also noticed that the learned model appears to be agnostic to species, seemingly demonstrating an ability of transfer learning. The availability of an explainable model that can consistently, rapidly, and accurately identify and quantify foliar stresses would have significant implications in scientific research, plant breeding, and crop production. The trained model could be deployed in mobile platforms (e.g., unmanned air vehicles and automated ground scouts) for rapid, large-scale scouting or as a mobile application for real-time detection of stress by farmers and researchers.
Available at: http://works.bepress.com/asheesh-singh/29/
This article is published as Ghosal, Sambuddha, David Blystone, Asheesh K. Singh, Baskar Ganapathysubramanian, Arti Singh, and Soumik Sarkar. "An explainable deep machine vision framework for plant stress phenotyping." Proceedings of the National Academy of Sciences (2018): 201716999. DOI: 10.1073/pnas.1716999115