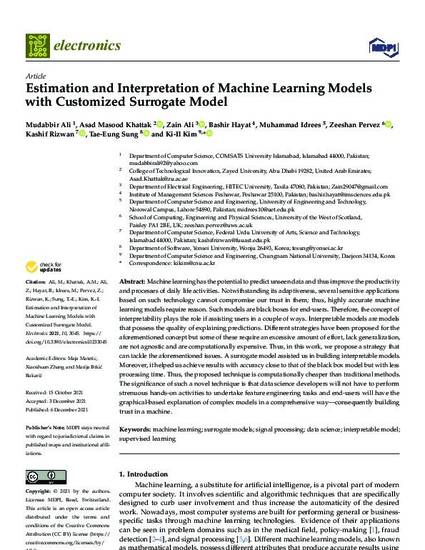
Machine learning has the potential to predict unseen data and thus improve the productivity and processes of daily life activities. Notwithstanding its adaptiveness, several sensitive applications based on such technology cannot compromise our trust in them; thus, highly accurate machine learning models require reason. Such models are black boxes for end-users. Therefore, the concept of interpretability plays the role if assisting users in a couple of ways. Interpretable models are models that possess the quality of explaining predictions. Different strategies have been proposed for the aforementioned concept but some of these require an excessive amount of effort, lack generalization, are not agnostic and are computationally expensive. Thus, in this work, we propose a strategy that can tackle the aforementioned issues. A surrogate model assisted us in building interpretable models. Moreover, it helped us achieve results with accuracy close to that of the black box model but with less processing time. Thus, the proposed technique is computationally cheaper than traditional methods. The significance of such a novel technique is that data science developers will not have to perform strenuous hands-on activities to undertake feature engineering tasks and end-users will have the graphical-based explanation of complex models in a comprehensive way—consequently building trust in a machine.
- Data science,
- Interpretable model,
- Machine learning,
- Signal processing,
- Supervised learning,
- Surrogate models
Available at: http://works.bepress.com/asad-khattak/104/