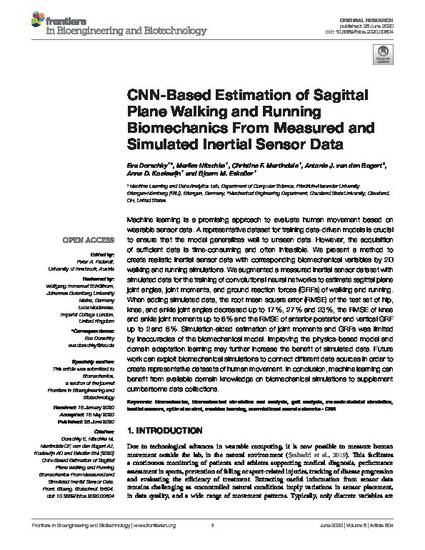
Machine learning is a promising approach to evaluate human movement based on wearable sensor data. A representative dataset for training data-driven models is crucial to ensure that the model generalizes well to unseen data. However, the acquisition of sufficient data is time-consuming and often infeasible. We present a method to create realistic inertial sensor data with corresponding biomechanical variables by 2D walking and running simulations. We augmented a measured inertial sensor dataset with simulated data for the training of convolutional neural networks to estimate sagittal plane joint angles, joint moments, and ground reaction forces (GRFs) of walking and running. When adding simulated data, the root mean square error (RMSE) of the test set of hip, knee, and ankle joint angles decreased up to 17 %, 27 % and 23 %, the RMSE of knee and ankle joint moments up to 6 % and the RMSE of anterior-posterior and vertical GRF up to 2 and 6 %. Simulation-aided estimation of joint moments and GRFs was limited by inaccuracies of the biomechanical model. Improving the physics-based model and domain adaptation learning may further increase the benefit of simulated data. Future work can exploit biomechanical simulations to connect different data sources in order to create representative datasets of human movement. In conclusion, machine learning can benefit from available domain knowledge on biomechanical simulations to supplement cumbersome data collections.
Available at: http://works.bepress.com/antonie_vandenbogert/120/