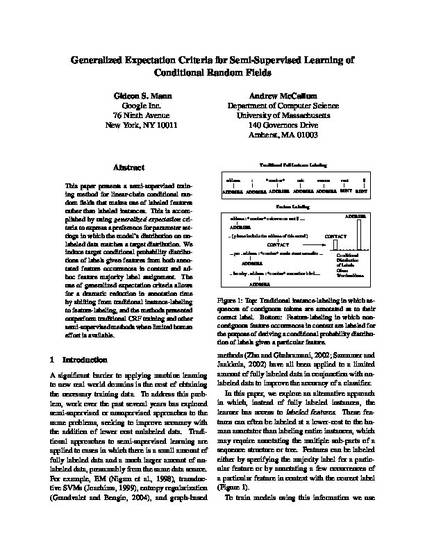
Unpublished Paper
Generalized Expectation Criteria for Semi-Supervised Learning of Conditional Random Fields
(2008)
Abstract
This paper presents a semi-supervised training method for linear-chain conditional random fields that makes use of labeled features rather than labeled instances. This is accomplished by using generalized expectation criteria to express a preference for parameter settings in which the model's distribution on unlabeled data matches a target distribution. We induce target conditional probability distributions of labels given features from both annotated feature occurrences in context and adhoc feature majority label assignment. The use of generalized expectation criteria allows for a dramatic reduction in annotation time by shifting from traditional instance-labeling to feature-labeling, and the methods presented outperform traditional CRF training and other semi-supervised methods when limited human effort is available.
Disciplines
Publication Date
2008
Comments
This is the pre-published version harvested from CIIR.
Citation Information
Gideon S. Mann and Andrew McCallum. "Generalized Expectation Criteria for Semi-Supervised Learning of Conditional Random Fields" (2008) Available at: http://works.bepress.com/andrew_mccallum/93/