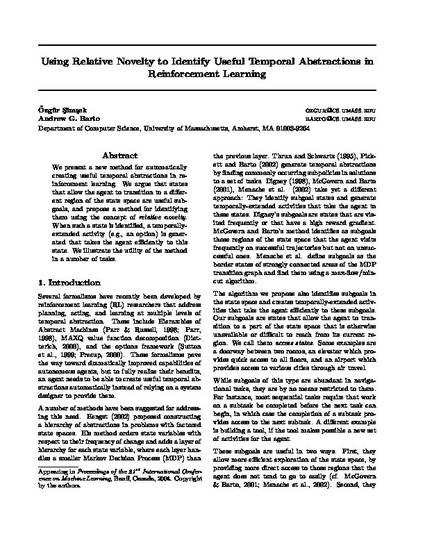
Other
Using Relative Novelty to Identify Useful Temporal Abstractions in Reinforcement Learning
Computer Science Department Faculty Publication Series
Publication Date
2004
Abstract
We present a new method for automatically creating useful temporal abstractions in reinforcement learning. We argue that states that allow the agent to transition to a different region of the state space are useful subgoals, and propose a method for identifying them using the concept of relative novelty. When such a state is identified, a temporallyextended activity (e.g., an option) is generated that takes the agent efficiently to this state. We illustrate the utility of the method in a number of tasks.
Disciplines
Comments
This paper was harvested from CiteSeer
Citation Information
Özgür Şimşek and Andrew G. Barto. "Using Relative Novelty to Identify Useful Temporal Abstractions in Reinforcement Learning" (2004) Available at: http://works.bepress.com/andrew_barto/3/