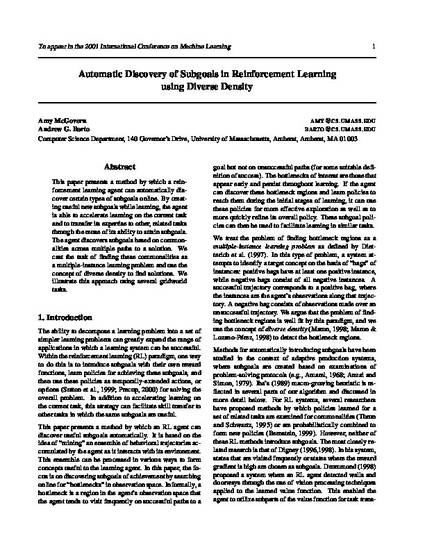
Other
Automatic Discovery of Subgoals in Reinforcement Learning using Diverse Density
Computer Science Department Faculty Publication Series
Publication Date
2001
Abstract
This paper presents a method by which a reinforcement learning agent can automatically discover certain types of subgoals online. By creating useful new subgoals while learning, the agent is able to accelerate learning on the current task and to transfer its expertise to other, related tasks through the reuse of its ability to attain subgoals. The agent discovers subgoals based on commonalities across multiple paths to a solution. We cast the task of finding these commonalities as a multiple-instance learning problem and use the concept of diverse density to find solutions. We illustrate this approach using several gridworld tasks.
Disciplines
Comments
This paper was harvested from CiteSeer
Citation Information
Amy McGovern and Andrew G. Barto. "Automatic Discovery of Subgoals in Reinforcement Learning using Diverse Density" (2001) Available at: http://works.bepress.com/andrew_barto/10/