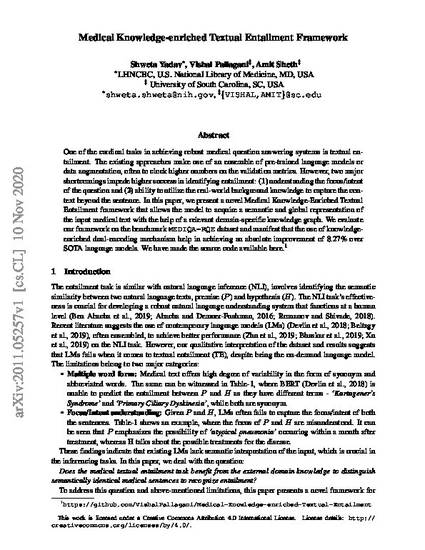
One of the cardinal tasks in achieving robust medical question answering systems is textual entailment. The existing approaches make use of an ensemble of pre-trained language models or data augmentation, often to clock higher numbers on the validation metrics. However, two major shortcomings impede higher success in identifying entailment: (1) understanding the focus/intent of the question and (2) ability to utilize the real-world background knowledge to capture the context beyond the sentence. In this paper, we present a novel Medical Knowledge-Enriched Textual Entailment framework that allows the model to acquire a semantic and global representation of the input medical text with the help of a relevant domain-specific knowledge graph. We evaluate our framework on the benchmark MEDIQA-RQE dataset and manifest that the use of knowledge enriched dual-encoding mechanism help in achieving an absolute improvement of 8.27% over SOTA language models. We have made the source code available here.
Yadav, S., Pallagani, V., & Sheth, A. (2020). Medical Knowledge-enriched Textual Entailment Framework. ArXiv:2011.05257 [Cs]. http://arxiv.org/abs/2011.05257
Available at: http://works.bepress.com/amit_sheth/640/