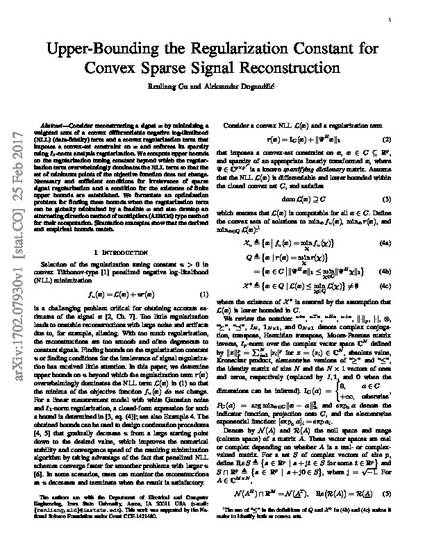
Consider reconstructing a signal x by minimizing a weighted sum of a convex differentiable negative log-likelihood (NLL) (data-fidelity) term and a convex regularization term that imposes a convex-set constraint on x and enforces its sparsity using ℓ1-norm analysis regularization. We compute upper bounds on the regularization tuning constant beyond which the regularization term overwhelmingly dominates the NLL term so that the set of minimum points of the objective function does not change. Necessary and sufficient conditions for irrelevance of sparse signal regularization and a condition for the existence of finite upper bounds are established. We formulate an optimization problem for finding these bounds when the regularization term can be globally minimized by a feasible x and also develop an alternating direction method of multipliers (ADMM) type method for their computation. Simulation examples show that the derived and empirical bounds match.
Available at: http://works.bepress.com/aleksandar_dogandzic/54/
This is the pre-print of the article Gu, Renliang, and Aleksandar Dogandžić. "Upper-Bounding the Regularization Constant for Convex Sparse Signal Reconstruction." arXiv preprint arXiv:1702.07930 (2017).