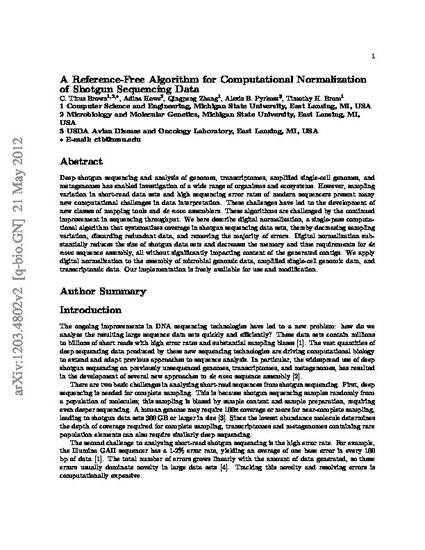
Unpublished Paper
A Reference-Free Algorithm for Computational Normalization of Shotgun Sequencing Data
arXiv:1203.4802
(2012)
Abstract
Deep shotgun sequencing and analysis of genomes, transcriptomes, amplified single-cell genomes, and metagenomes has enabled investigation of a wide range of organisms and ecosystems. However, sampling variation in short-read data sets and high sequencing error rates of modern sequencers present many new computational challenges in data interpretation. These challenges have led to the development of new classes of mapping tools and {\em de novo} assemblers. These algorithms are challenged by the continued improvement in sequencing throughput. We here describe digital normalization, a single-pass computational algorithm that systematizes coverage in shotgun sequencing data sets, thereby decreasing sampling variation, discarding redundant data, and removing the majority of errors. Digital normalization substantially reduces the size of shotgun data sets and decreases the memory and time requirements for {\em de novo} sequence assembly, all without significantly impacting content of the generated contigs. We apply digital normalization to the assembly of microbial genomic data, amplified single-cell genomic data, and transcriptomic data. Our implementation is freely available for use and modification.
Disciplines
Publication Date
May 21, 2012
Comments
Licensed under a CC BY license.
Citation Information
C. Titus Brown, Adina Howe, Qingpeng Zhang, Alexis B. Pyrkosz, et al.. "A Reference-Free Algorithm for Computational Normalization of Shotgun Sequencing Data" arXiv:1203.4802 (2012) Available at: http://works.bepress.com/adina/10/