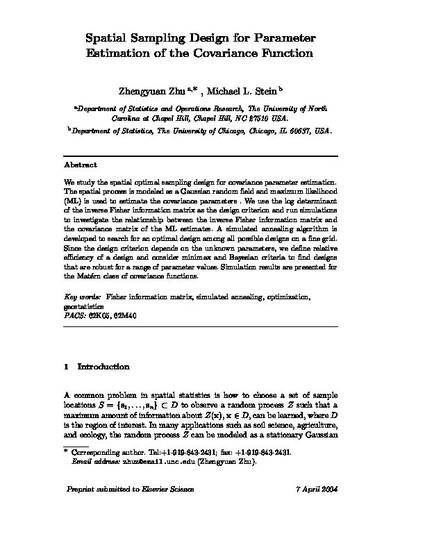
Article
Spatial sampling design for parameter estimation of the covariance function
Journal of Statistical Planning and Inference
(2005)
Abstract
We study the spatial optimal sampling design for covariance parameter estimation. The spatial process is modeled as a Gaussian random field and maximum likelihood (ML) is used to estimate the covariance parameters. We use the log determinant of the inverse Fisher information matrix as the design criterion and run simulations to investigate the relationship between the inverse Fisher information matrix and the covariance matrix of the ML estimates. A simulated annealing algorithm is developed to search for an optimal design among all possible designs on a fine grid. Since the design criterion depends on the unknown parameters, we define relative efficiency of a design and consider minimax and Bayesian criteria to find designs that are robust for a range of parameter values. Simulation results are presented for the Matérn class of covariance functions.
Keywords
- Fisher information matrix,
- Simulated annealing,
- Optimization,
- Geostatistics
Disciplines
Publication Date
October 1, 2005
DOI
10.1016/j.jspi.2004.04.017
Publisher Statement
This is a manuscript of an article published as Zhu, Zhengyuan, and Michael L. Stein. "Spatial sampling design for parameter estimation of the covariance function." Journal of Statistical Planning and Inference 134, no. 2 (2005): 583-603. Copyright 2005 Elsevier B.V.
Citation Information
Zhengyuan Zhu and Michael L. Stein. "Spatial sampling design for parameter estimation of the covariance function" Journal of Statistical Planning and Inference Vol. 134 Iss. 2 (2005) p. 583 - 603 Available at: http://works.bepress.com/zhengyuan-zhu/8/
Creative Commons license

This work is licensed under a Creative Commons CC_BY-NC-ND International License.