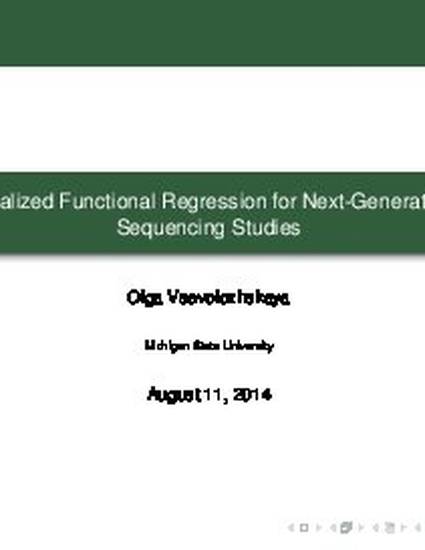
Advances in next-generation sequencing (NGS) technologies make it possible to examining nearly every variant on the human genome. Because of the high density of variants, genotypes within a genetic region can be thought of as a finite sample realization of some underlying stochastic process. The aim of vast majority of NSG studies is to explore an association between a set of genetic variants and qualitative/quantitative traits. To address this aim, we propose a general framework based on functional dependent variable and univariate covariates. We fit our model using penalized least squares criterion. We note that within this framework, the spline estimates evaluated at design points (i.e., the observation genomic positions) are the same as the Best Linear Unbiased Predictor (BLUP) estimates of a linear mixed model. By exploiting this connection to mixed models, our approach allows a broad testing framework for an association between a genotype function and multiple traits. We conduct a simulation study to better understand advantages and disadvantages of our approach compared to existing methods. We illustrate our methodology by applying it to data from Dallas Heart Study.
- rare variants,
- functional data analysis,
- functional regression model,
- penalized likelihood,
- functional response,
- multiple predictors
Available at: http://works.bepress.com/vsevolozhskaya/20/