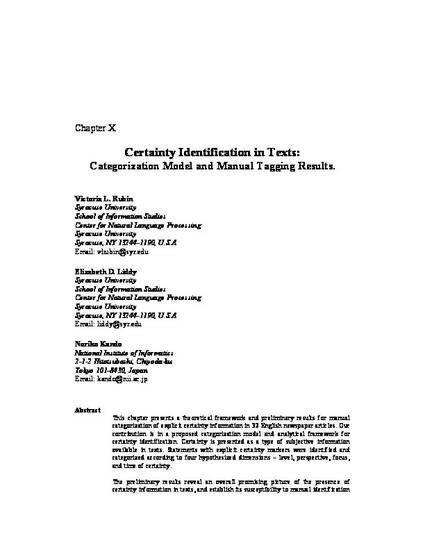
- certainty,
- certainty identification,
- certainty categorization model,
- subjectivity,
- manual tagging,
- natural language processing,
- linguistics,
- information extraction,
- information retrieval; uncertainty,
- doubt,
- epistemic comments,
- evidentials,
- hedges,
- hedging,
- certainty expressions; levels of certainty,
- point of view,
- annotating opinions; newspaper article analysis,
- analysis of editorials
This chapter presents a theoretical framework and preliminary results for manual categorization of explicit certainty information in 32 English newspaper articles. Our contribution is in a proposed categorization model and analytical framework for certainty identification. Certainty is presented as a type of subjective information available in texts. Statements with explicit certainty markers were identified and categorized according to four hypothesized dimensions – level, perspective, focus, and time of certainty.
The preliminary results reveal an overall promising picture of the presence of certainty information in texts, and establish its susceptibility to manual identification within the proposed four-dimensional certainty categorization analytical framework. Our findings are that the editorial sample group had a significantly higher frequency of markers per sentence than did the sample group of news stories. For editorials, high level of certainty, writer’s point of view, and future and present time were the most populated categories. For news stories, the most common were high and moderate levels, directly involved third party’s point of view, and past time. These patterns have positive practical implications for automation.