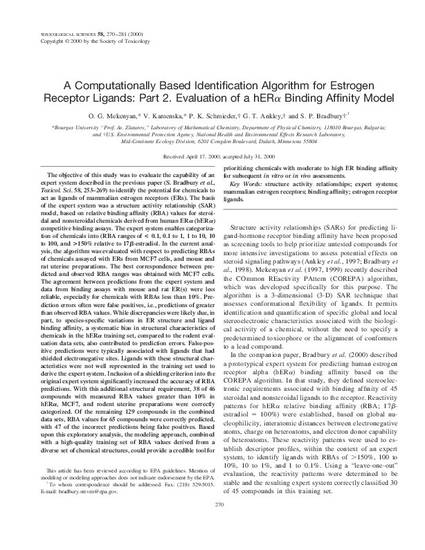
The objective of this study was to evaluate the capability of an expert system described in the previous paper (S. Bradbury et al., Toxicol. Sci. 58, 253–269) to identify the potential for chemicals to act as ligands of mammalian estrogen receptors (ERs). The basis of the expert system was a structure activity relationship (SAR) model, based on relative binding affinity (RBA) values for steroidal and nonsteroidal chemicals derived from human ERa (hERa) competitive binding assays. The expert system enables categorization of chemicals into (RBA ranges of < 0.1, 0.1 to 1, 1 to 10, 10 to 100, and >150% relative to 17b-estradiol. In the current analysis, the algorithm was evaluated with respect to predicting RBAs of chemicals assayed with ERs from MCF7 cells, and mouse and rat uterine preparations. The best correspondence between predicted and observed RBA ranges was obtained with MCF7 cells. The agreement between predictions from the expert system and data from binding assays with mouse and rat ER(s) were less reliable, especially for chemicals with RBAs less than 10%. Prediction errors often were false positives, i.e., predictions of greater than observed RBA values. While discrepancies were likely due, in part, to species-specific variations in ER structure and ligand binding affinity, a systematic bias in structural characteristics of chemicals in the hERa training set, compared to the rodent evaluation data sets, also contributed to prediction errors. False-positive predictions were typically associated with ligands that had shielded electronegative sites. Ligands with these structural characteristics were not well represented in the training set used to derive the expert system. Inclusion of a shielding criterion into the original expert system significantly increased the accuracy of RBA predictions. With this additional structural requirement, 38 of 46 compounds with measured RBA values greater than 10% in hERa, MCF7, and rodent uterine preparations were correctly categorized. Of the remaining 129 compounds in the combined data sets, RBA values for 65 compounds were correctly predicted, with 47 of the incorrect predictions being false positives. Based upon this exploratory analysis, the modeling approach, combined with a high-quality training set of RBA values derived from a diverse set of chemical structures, could provide a credible tool for prioritizing chemicals with moderate to high ER binding affinity for subsequent in vitro or in vivo assessments.
- structure activity relationships;,
- expert systems,
- mammalian estrogen receptors,
- binding affinity,
- estrogen receptor ligands
Available at: http://works.bepress.com/steven_bradbury/13/