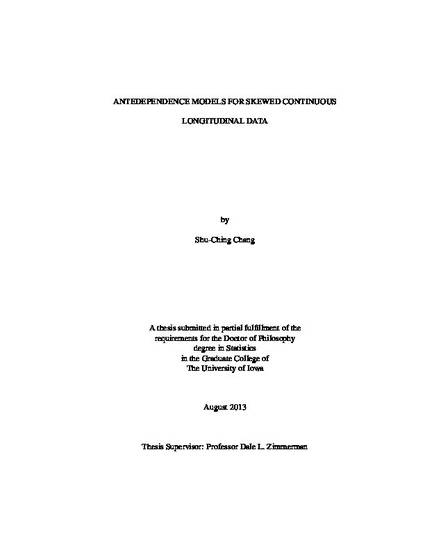
This thesis explores the problems of fitting antedependence (AD) models and partial antecorrelation (PAC) models to continuous non-Gaussian longitudinal data. AD models impose certain conditional independence relations among the measurements within each subject, while PAC models characterize the partial correlation relations. The models are parsimonious and useful for data exhibiting time-dependent correlations.
Since the relation of conditional independence among variables is rather restrictive, we first consider an autoregressively characterized PAC model with independent asymmetric Laplace (ALD) innovations and prove that this model is an AD model. The ALD distribution previously has been applied to quantile regression and has shown promise for modeling asymmetrically distributed ecological data. In addition, the double exponential distribution, a special case of the ALD, has played an important role in fitting symmetric finance and hydrology data. We give the distribution of a linear combination of independent standard ALD variables in order to derive marginal distributions for the model. For the model estimation problem, we propose an iterative algorithm for the maximum likelihood estimation. The estimation accuracy is illustrated by some numerical examples as well as some longitudinal data sets.
The second component of this dissertation focuses on AD multivariate skew normal models. The multivariate skew normal distribution not only shares some nice properties with multivariate normal distributions but also allows for any value of skewness. We derive necessary and sufficient conditions on the shape and covariance parameters for multivariate skew normal variables to be AD(p) for some p. Likelihood-based estimation for balanced and monotone missing data as well as likelihood ratio hypothesis tests for the order of antedependence and for zero skewness under the models are presented.
Since the class of skew normal random variables is closed under the addition of independent standard normal random variables, we then consider an autoregressively characterized PAC model with a combination of independent skew normal and normal innovations. Explicit expressions for the marginals, which all have skew normal distributions, and maximum likelihood estimates of model parameters, are given.
Numerical results show that these three proposed models may provide reasonable fits to some continuous non-Gaussian longitudinal data sets. Furthermore, we compare the fits of these models to the Treatment A cattle growth data using penalized likelihood criteria, and demonstrate that the AD(2) multivariate skew normal model fits the data best among those proposed models.
- Antedependence,
- Asymmetric Laplace Distribution,
- Likelihood-Based Estimation,
- Longitudinal Data Analysis,
- Partial Antecorrelation,
- Skew Normal Distribution
Available at: http://works.bepress.com/shu-ching-chang/8/