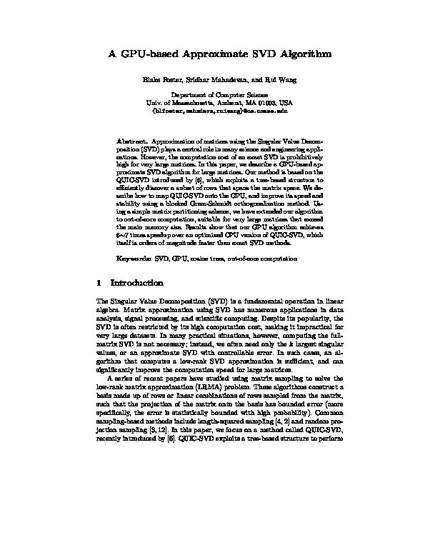
Unpublished Paper
A GPU-based Approximate SVD Algorithm
Lecture Notes in Computer Science
(2012)
Abstract
Approximation of matrices using the Singular Value Decomposition (SVD) plays a central role in many science and engineering applications. However, the computation cost of an exact SVD is prohibitively high for very large matrices. In this paper, we describe a GPU-based approximate SVD algorithm for large matrices. Our method is based on the QUIC-SVD introduced by [6], which exploits a tree-based structure to efficiently discover a subset of rows that spans the matrix space. We describe how to map QUIC-SVD onto the GPU, and improve its speed and stability using a blocked Gram-Schmidt orthogonalization method. Using a simple matrix partitioning scheme, we have extended our algorithm to out-of-core computation, suitable for very large matrices that exceed the main memory size. Results show that our GPU algorithm achieves 6~7 times speedup over an optimized CPU version of QUIC-SVD, which itself is orders of magnitude faster than exact SVD methods.
Keywords
- SVD,
- GPU,
- Cosine Trees,
- Out-of-core computation
Disciplines
Publication Date
2012
Comments
Published version is located at http://link.springer.com/chapter/10.1007%2F978-3-642-31464-3_58
Citation Information
Blake Foster, Sridhar Mahadevan and Rui Wang. "A GPU-based Approximate SVD Algorithm" Lecture Notes in Computer Science (2012) Available at: http://works.bepress.com/rui_wang/2/