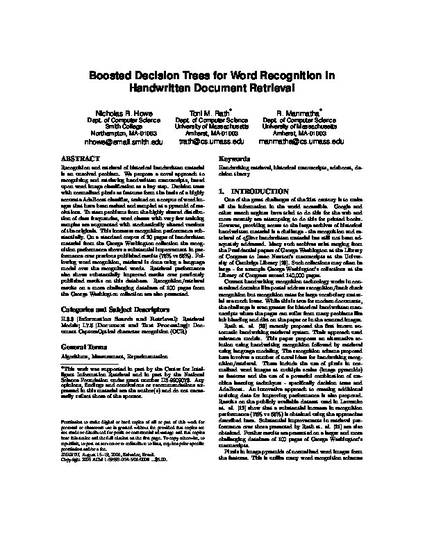
Recognition and retrieval of historical handwritten material is an unsolved problem. We propose a novel approach to recognizing and retrieving handwritten manuscripts, based upon word image classification as a key step. Decision trees with normalized pixels as features form the basis of a highly accurate AdaBoost classifier, trained on a corpus of word images that have been resized and sampled at a pyramid of resolutions. To stem problems from the highly skewed distribution of class frequencies, word classes with very few training samples are augmented with stochastically altered versions of the originals. This increases recognition performance substantially. On a standard corpus of 20 pages of handwritten material from the George Washington collection the recognition performance shows a substantial improvement in performance over previous published results (75% vs 65%). Following word recognition, retrieval is done using a language model over the recognized words. Retrieval performance also shows substantially improved results over previously published results on this database. Recognition/retrieval results on a more challenging database of 100 pages from the George Washington collection are also presented.
- Information Search and Retrieval,
- Document and Text Processing,
- Handwriting retrieval,
- historical manuscripts,
- adaboost,
- decision theory
Available at: http://works.bepress.com/r_manmatha/36/