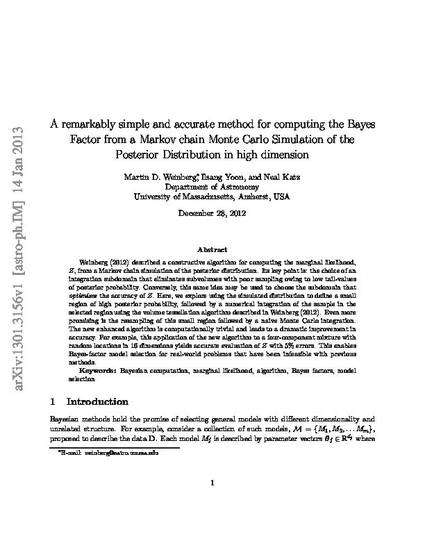
Unpublished Paper
A Remarkably Simple and Accurate Method for Computing the Bayes Factor from a Markov Chain Monte Carlo Simulation of the Posterior Distribution in High Dimension
Bayesian Analysis
(2012)
Abstract
Weinberg (2012) described a constructive algorithm for computing the marginal likelihood, Z, from a Markov chain simulation of the posterior distribution. Its key point is: the choice of an integration subdomain that eliminates subvolumes with poor sampling owing to low tail-values of posterior probability. Conversely, this same idea may be used to choose the subdomain that optimizes the accuracy of Z. Here, we explore using the simulated distribution to define a small region of high posterior probability, followed by a numerical integration of the sample in the selected region using the volume tessellation algorithm described inWeinberg (2012). Even more promising is the resampling of this small region followed by a naive Monte Carlo integration. The new enhanced algorithm is computationally trivial and leads to a dramatic improvement in accuracy. For example, this application of the new algorithm to a four-component mixture with random locations in 16 dimensions yields accurate evaluation of Z with 5% errors. This enables Bayes-factor model selection for real-world problems that have been infeasible with previous methods.
Keywords
- Bayesian computation,
- marginal likelihood,
- algorithm,
- Bayes factor,
- model selection
Disciplines
Publication Date
2012
Comments
Prepublished version downloaded from ArXiv.
Citation Information
Martin D. Weinberg, Ilsang Yoon and Neal S. Katz. "A Remarkably Simple and Accurate Method for Computing the Bayes Factor from a Markov Chain Monte Carlo Simulation of the Posterior Distribution in High Dimension" Bayesian Analysis (2012) Available at: http://works.bepress.com/neal_katz/12/