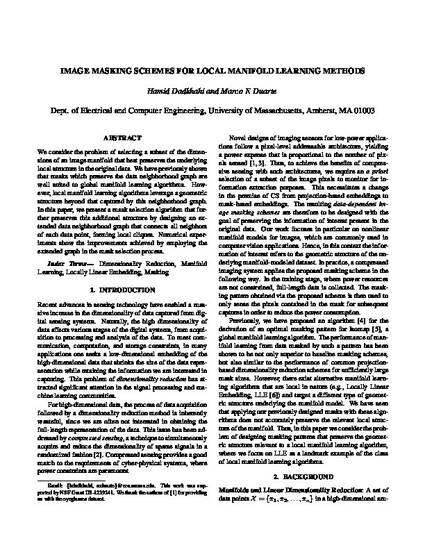
Presentation
Image Masking Schemes for Local Manifold Learning Methods
IEEE International Conference on Acoustics, Speech, and Signal Processing (ICASSP)
(2015)
Abstract
We consider the problem of selecting a subset of the dimensions of an image manifold that best preserves the underlying local structure in the original data. We have previously shown that masks which preserve the data neighborhood graph are well suited to global manifold learning algorithms. However, local manifold learning algorithms leverage a geometric structure beyond that captured by this neighborhood graph. In this paper, we present a mask selection algorithm that further preserves this additional structure by designing an extended data neighborhood graph that connects all neighbors of each data point, forming local cliques. Numerical experiments show the improvements achieved by employing the extended graph in the mask selection process.
Disciplines
Publication Date
2015
Comments
This is an author manuscript of this conference paper. More information about the conference can be found at http://icassp2015.org/
Citation Information
Hamid Dadkhahi and Marco Duarte. "Image Masking Schemes for Local Manifold Learning Methods" IEEE International Conference on Acoustics, Speech, and Signal Processing (ICASSP) (2015) Available at: http://works.bepress.com/marco_duarte/9/