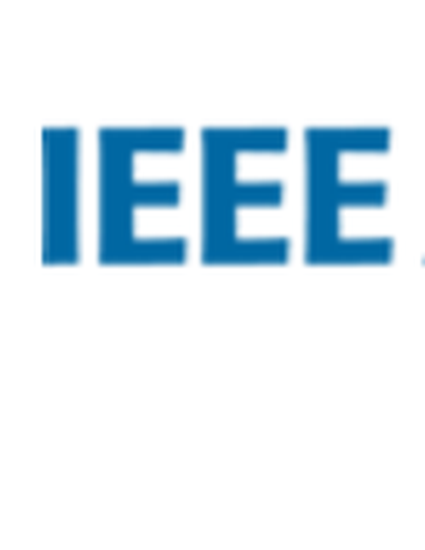
Spike-timing-dependent plasticity (STDP) is a fundamental synaptic learning rule observed in biology that leads to numerous behavioral and cognitive outcomes. Emulating STDP in electronic spiking neural networks with high-density memristive synapses is, therefore, of significant interest. While one popular method involves pulse-shaping the spiking neuron output voltages, an alternative approach is outlined in this article. The proposed STDP implementation uses time-varying dynamic resistance [R(t)] elements to achieve local synaptic learning from spike-pair STDP, spike triplet STDP, and firing rates. The R(t) elements are connected to each neuron circuit, thereby maintaining synaptic density and leveraging voltage division as a means of altering synaptic weight (memristor voltage). Example R(t) elements with their corresponding behaviors are demonstrated through simulation. A three-input-two-output network using single-memristor synaptic connections and R(t) elements is also simulated. Network-level effects, such as nonspecific synaptic plasticity, are discussed. Finally, spatiotemporal pattern recognition (STPR) using R(t) elements is demonstrated in simulation.
Available at: http://works.bepress.com/kurtis_cantley/36/