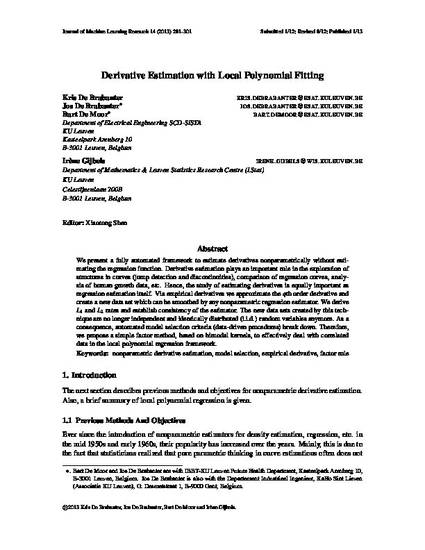
We present a fully automated framework to estimate derivatives nonparametrically without estimating the regression function. Derivative estimation plays an important role in the exploration of structures in curves (jump detection and discontinuities), comparison of regression curves, analysis of human growth data, etc. Hence, the study of estimating derivatives is equally important as regression estimation itself. Via empirical derivatives we approximate the qth order derivative and create a new data set which can be smoothed by any nonparametric regression estimator. We derive L1 and L2 rates and establish consistency of the estimator. The new data sets created by this technique are no longer independent and identically distributed (i.i.d.) random variables anymore. As a consequence, automated model selection criteria (data-driven procedures) break down. Therefore, we propose a simple factor method, based on bimodal kernels, to effectively deal with correlated data in the local polynomial regression framework.
- empirical derivative,
- factor rule,
- model selection,
- nonparametric derivative estimation
Available at: http://works.bepress.com/kris_debrabanter/1/