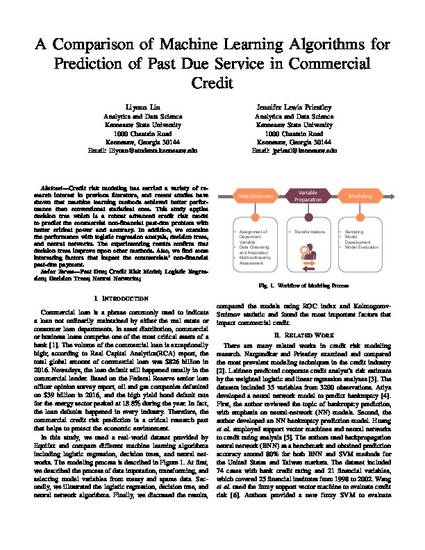
Article
A Comparison of Machine Learning Algorithms for Prediction of Past Due Service in Commercial Credit
Published and Grey Literature from PhD Candidates
Department
Statistics and Analytical Sciences
Document Type
Article
Submission Date
4-1-2018
Disciplines
Abstract
Credit risk modeling has carried a variety of research interest in previous literature, and recent studies have shown that machine learning methods achieved better performance than conventional statistical ones. This study applies decision tree which is a robust advanced credit risk model to predict the commercial non-financial past-due problem with better critical power and accuracy. In addition, we examine the performance with logistic regression analysis, decision trees, and neural networks. The experimenting results confirm that decision trees improve upon other methods. Also, we find some interesting factors that impact the commercials’ non-financial past-due payment.
Citation Information
Liyuan Liu and Jennifer Lewis Priestley. "A Comparison of Machine Learning Algorithms for Prediction of Past Due Service in Commercial Credit" (2018) Available at: http://works.bepress.com/jennifer_priestley/37/