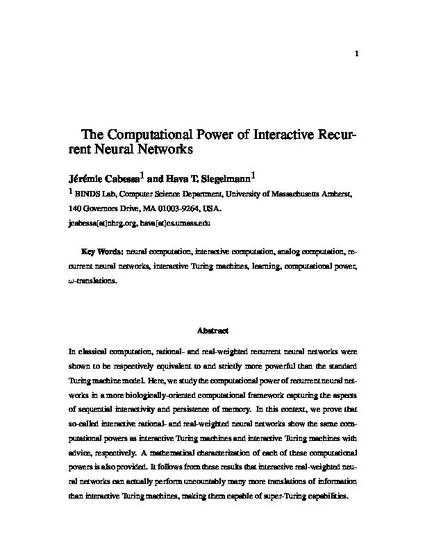
Article
The Computational Power of Interactive Recurrent Neural Networks
Neural Computation
(2012)
Abstract
In classical computation, rational- and real-weighted recurrent neural networks were shown to be respectively equivalent to and strictly more powerful than the standard Turing machine model. Here, we study the computational power of recurrent neural networks in a more biologically oriented computational framework, capturing the aspects of sequential interactivity and persistence of memory. In this context, we prove that so-called interactive rational- and real-weighted neural networks show the same computational powers as interactive Turing machines and interactive Turing machines with advice, respectively. A mathematical characterization of each of these computational powers is also provided. It follows from these results that interactive real-weighted neural networks can perform uncountably many more translations of information than interactive Turing machines, making them capable of super-Turing capabilities.
Keywords
- neural computation,
- interactive computation,
- analog computation,
- recurrent neural networks,
- interactive Turing machines,
- learning,
- computational power,
- w-translations
Disciplines
Publication Date
April, 2012
Publisher Statement
doi: 10.1162/NECO_a_00263
Citation Information
Jèrèmie Cabessa and Hava Siegelmann. "The Computational Power of Interactive Recurrent Neural Networks" Neural Computation Vol. 24 Iss. 4 (2012) Available at: http://works.bepress.com/hava_siegelmann/1/