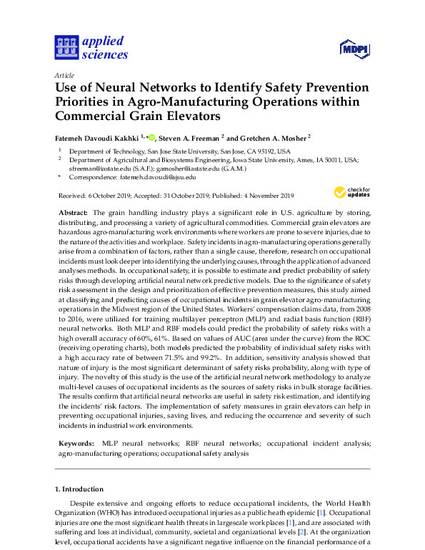
Article
Use of Neural Networks to Identify Safety Prevention Priorities in Agro-Manufacturing Operations within Commercial Grain Elevators
Applied Sciences
(2019)
Abstract
The grain handling industry plays a significant role in U.S. agriculture by storing, distributing, and processing a variety of agricultural commodities. Commercial grain elevators are hazardous agro-manufacturing work environments where workers are prone to severe injuries, due to the nature of the activities and workplace. Safety incidents in agro-manufacturing operations generally arise from a combination of factors, rather than a single cause, therefore, research on occupational incidents must look deeper into identifying the underlying causes, through the application of advanced analyses methods. In occupational safety, it is possible to estimate and predict probability of safety risks through developing artificial neural network predictive models. Due to the significance of safety risk assessment in the design and prioritization of effective prevention measures, this study aimed at classifying and predicting causes of occupational incidents in grain elevator agro-manufacturing operations in the Midwest region of the United States. Workers’ compensation claims data, from 2008 to 2016, were utilized for training multilayer perceptron (MLP) and radial basis function (RBF) neural networks. Both MLP and RBF models could predict the probability of safety risks with a high overall accuracy of 60%, 61%. Based on values of AUC (area under the curve) from the ROC (receiving operating charts), both models predicted the probability of individual safety risks with a high accuracy rate of between 71.5% and 99.2%. In addition, sensitivity analysis showed that nature of injury is the most significant determinant of safety risks probability, along with type of injury. The novelty of this study is the use of the artificial neural network methodology to analyze multi-level causes of occupational incidents as the sources of safety risks in bulk storage facilities. The results confirm that artificial neural networks are useful in safety risk estimation, and identifying the incidents’ risk factors. The implementation of safety measures in grain elevators can help in preventing occupational injuries, saving lives, and reducing the occurrence and severity of such incidents in industrial work environments.
Disciplines
Publication Date
Fall November 4, 2019
DOI
https://doi.org/10.3390/app9214690
Citation Information
Fatemeh Davoudi Kakhki, Steven A Freeman and Gretchen A. Mosher. "Use of Neural Networks to Identify Safety Prevention Priorities in Agro-Manufacturing Operations within Commercial Grain Elevators" Applied Sciences Vol. 9 Iss. 21 (2019) p. 4690 Available at: http://works.bepress.com/fatemeh-davoudikakhki/6/