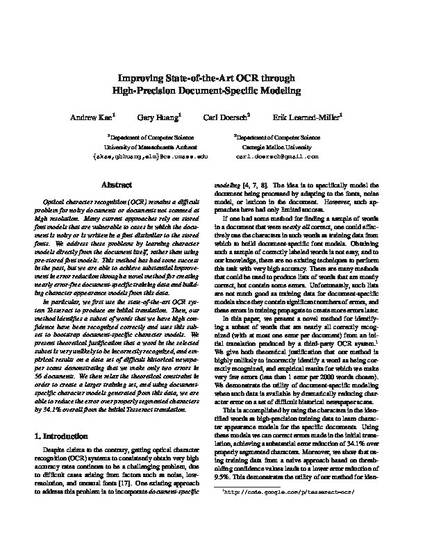
Optical character recognition (OCR) remains a difficult problem for noisy documents or documents not scanned at high resolution. Many current approaches rely on stored font models that are vulnerable to cases in which the docu- ment is noisy or is written in a font dissimilar to the stored fonts. We address these problems by learning character models directly from the document itself, rather than using pre-stored font models. This method has had some success in the past, but we are able to achieve substantial improve- ment in error reduction through a novel method for creating nearly error-free document-specifictraining data and buil d- ing character appearance models from this data. In particular, we first use the state-of-the-art OCR sys- tem Tesseract to produce an initial translation. Then, our method identifies a subset of words that we have high con- fidence have been recognized correctly and uses this sub- set to bootstrap document-specific character models. We present theoretical justification that a word in the selecte d subset is very unlikely to be incorrectly recognized, and em - pirical results on a data set of difficult historical newspa- per scans demonstrating that we make only two errors in 56 documents. We then relax the theoretical constraint in order to create a larger training set, and using document- specific character models generated from this data, we are able to reduce the error over properly segmented characters by 34.1% overall from the initial Tesseract translation.
Available at: http://works.bepress.com/erik_learned_miller/56/