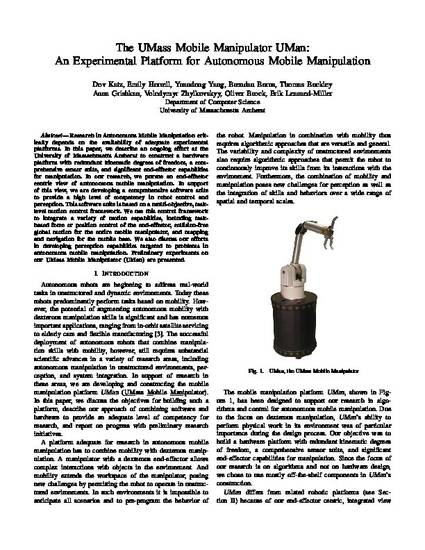
Object identification is the task of identifying specific objects belonging to the same class such as cars. We often need to recognize an object that we have only seen a few times. In fact, we often observe only one example of a particular object before we need to recognize it again. Thus we are interested in building a system which can learn to extract distinctive markers from a single example and which can then be used to identify the object in another image as “same ” or “different”. Previous work by Ferencz et al. introduced the notion of hyper-features, which are properties of an image patch that can be used to estimate the utility of the patch in subsequent matching tasks. In this work, we show that hyper-feature based models can be more efficiently estimated using discriminative training techniques. In particular, we describe a new hyper-feature model based upon logistic regression that shows improved performance over previously published techniques. Our approach significantly outperforms Bayesian face recognition that is considered as a standard benchmark for face recognition
Available at: http://works.bepress.com/erik_learned_miller/37/