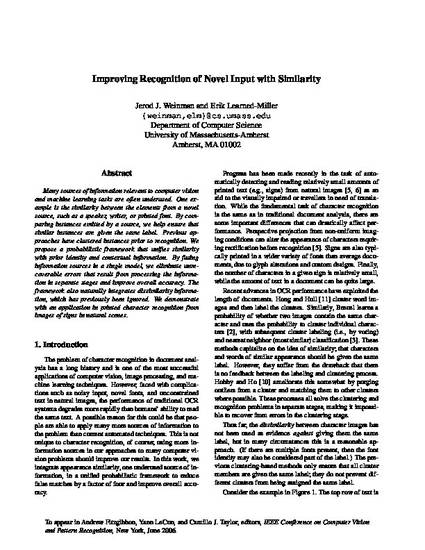
Many sources of information relevant to computer vision and machine learning tasks are often underused. One example is the similarity between the elements from a novel source, such as a speaker, writer, or printed font. By comparing instances emitted by a source, we help ensure that similar instances are given the same label. Previous approaches have clustered instances prior to recognition. We propose a probabilistic framework that unifies similarity with prior identity and contextual information. By fusing information sources in a single model, we eliminate unrecoverable errors that result from processing the information in separate stages and improve overall accuracy. The framework also naturally integrates dissimilarity information, which has previously been ignored. We demonstrate with an application in printed character recognition from images of signs in natural scenes.
Available at: http://works.bepress.com/erik_learned_miller/34/