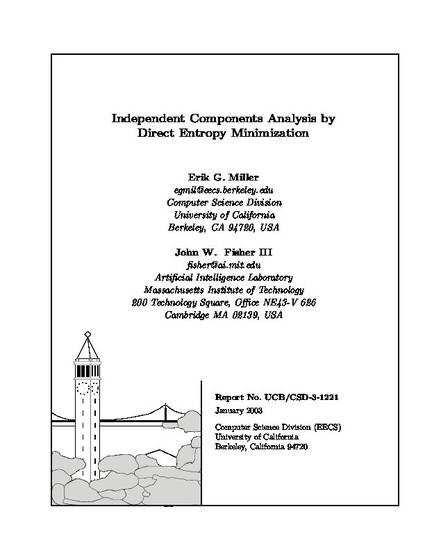
This paper presents a new algorithm for the independent components analysis (ICA) problem based on efficient entropy estimates. Like many previous methods, this algorithm directly minimizes the measure of departure from independence according to the estimated Kullback-Leibler divergence between the joint distribution and the product of the marginal distributions. We pair this approach with efficient entropy estimators from the statistics literature. In particular, the entropy estimator we use is consistent and exhibits rapid convergence. The algorithm based on this estimator is simple, computationally efficient, intuitively appealing, and outperforms other well known algorithms. In addition, the estimator's relative insensitivity to outliers translates into superior performance by our ICA algorithm on outlier tests. We present favorable comparisons to the Kernel ICA, FAST-ICA, JADE, and extended Infomax algorithms in extensive simulations.
Available at: http://works.bepress.com/erik_learned_miller/12/