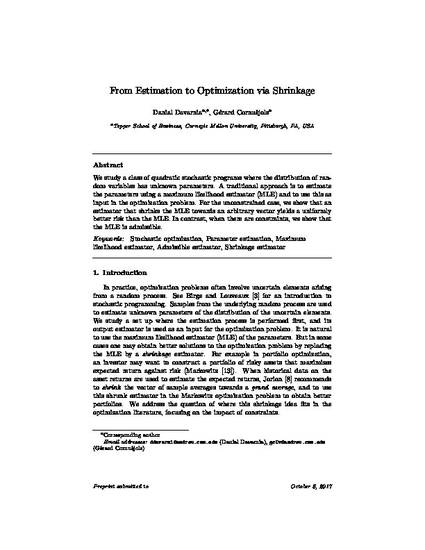
Article
From Estimation to Optimization via Shrinkage
Operations Research Letters
(2017)
Abstract
We study a class of quadratic stochastic programs where the distribution of random variables has unknown parameters. A traditional approach is to estimate the parameters using a maximum likelihood estimator (MLE) and to use this as input in the optimization problem. For the unconstrained case, we show that an estimator that shrinks the MLE towards an arbitrary vector yields a uniformly better risk than the MLE. In contrast, when there are constraints, we show that the MLE is admissible.
Keywords
- Stochastic optimization,
- Parameter estimation,
- Maximum likelihood estimator,
- Admissible estimator,
- Shrinkage estimator
Disciplines
Publication Date
November, 2017
DOI
https://doi.org/10.1016/j.orl.2017.10.005
Publisher Statement
© 2017 Elsevier B.V. All rights reserved.
Citation Information
Danial Davarnia and Gerard Cornuejols. "From Estimation to Optimization via Shrinkage" Operations Research Letters Vol. 45 Iss. 6 (2017) p. 642 - 646 Available at: http://works.bepress.com/danial-davarnia/1/